Towards Machine Learning for Acoustic Resonance Technology
FT wind sensors measure wind speed and direction using an acoustic field superimposed on a flow field in an acoustic resonator equipped with three piezoelectric transducers. The study proposed here focuses on the acoustic resonance technology of a simplified wind sensor geometry, and how it is affected by geometrical tolerances. In particular, it will explore the advantages of using machine learning algorithms to save computational costs without compromising the accuracy and reliability of the results.
Due to the complexity of the interactions between the acoustics field and the flow, decoupling them through Finite Elements simulations is essential to better understand the behaviour of the sensor. However, the numerical simulations must be properly defined to guarantee the accuracy of the results; therefore, the first step of this study will propose the setup and validation of an FE simulation to study the acoustic field of the wind sensor in the absence of flow.
The simplified geometry of the wind sensor, illustrated in Fig.1, is imported in COMSOL Multiphysics® with the LiveLink for Solidworks®, which will be thoroughly exploited for this study. The computational domain is set up following an optimisation study outlined in reference [1], and it consists of a block that encompasses half of the resonator area and expands half wavelength outside of it, as shown in Fig.2. The entire computational domain is composed exclusively of air, with the sensor’s walls treated as boundary conditions with an optimised absorption coefficient. The pressure acoustic physics is applied to the whole domain as this study will focus on the acoustic response of the resonator in the absence of flow in the frequency domain. The sensor’s behaviour is replicated treating the transmitting transducer (B following the nomenclature in Fig.2) as a piston oscillating with constant speed amplitude across the frequencies of interest and measuring the signal on transducer A.
The results of this simulation setup have already been validated against experimental data. In particular, the normalised pressure magnitude on the receiving transducer (A) was compared with the voltage signal received by the real transducer while testing, and the results are reported in Fig.3.
After the validation, this study proposes to create a surrogate model, exploiting the new functionality introduced by COMSOL® v6.2 to vary 10 geometrical parameters and be able to assess their effects on the acoustic response in the absence of flow, without the need to conduct FE simulations. The surrogate model is set up using as input parameters the geometrical features which are varied in their tolerances limit with a normal distribution around the nominal value and the frequency which is varied uniformly in the range of interest.
The outcome of this analysis is to optimise the number of points needed in the Design of Experiments (DoE) table, the structure of the Deep Neural Network (DNN), the loss function used, and the duration of the DNN training. The results will focus on the comparison between the surrogate modelling and FE simulations in terms of accuracy, reliability, and computational time.
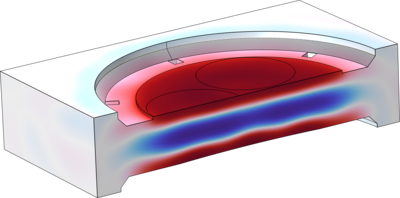
Herunterladen
- paper_comsol-conference-2024-florence.pdf - 0.82MB
- merico_10791_poster.pdf - 2.02MB
- 2_elisabetta_merico.pdf - 1.39MB