Iterative Learning Control for Spatio-Temporal Repetitive Processes
Recently, due to the dynamically increasing complexity of modern systems, a strong necessity appears for more systematic approaches to high quality control and process monitoring. Requirements imposed by process control in the area of spatio-temporal physical systems also called distributed parameter systems (DPSs) are associated with using very accurate models in which spatial dynamics cannot be neglected and has to be included in addition to the temporal one. Many classical control approaches dedicated for lumped parameter systems are not able to meet these sophisticated requirements.
In the case of repetitive processes it can be observed that in each replicated trial, the system produce at the output the same tracking error, oscillations and overshot, and that characteristic could be used to improvement of tracking performance. For that problems, a promising approach is constituted by technique called Iterative Learning Control (ILC), which since the late 70s [1][2] has established a separate field of control theory. The learning convergence of ILC is guaranteed through rigorous analysis, without any simplification or discretization of the dynamics in time, space as well as iteration domains [3].
As an example of repetitive process, a combustion process has been modeled using COMSOL Multiphysics® software. In particular, the Navier-Stokes equations for fluid dynamics, together with diffusion and convection were used to simulate gas transport inside the reactor. General feedback control scheme was developed for tracking reference signal inside the reactor to get high performance of control process. In addition to this the optimal location of sensor measuring the system state is considered in order to provide the most informative data for the process identification. The main aim of this work is to provide a strong evidence that ILC considered to be efficient tool for lumped systems could be successfully adopted for DPSs with restrictions according to control signal, quality of tracking etc. Finally, it will be compared with other control approaches enabling the controlling similar process.
The preliminary results are presented in Fig.1 where reference and final time profile for concentration is shown. In Fig. 2 the errors in first and last trial are presented and in Fig. 3 illustrates the convergence of the error norm for all trials on log scale.
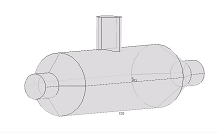
Herunterladen
- kowalów_presentation.pdf - 1.44MB
- kowalów_paper.pdf - 0.42MB
- kowalów_abstract.pdf - 0.13MB